Abstract
When we use language, we usually assume that the meaning of our statements is clear and that others can understand precisely this meaning. However, that this may not always be the case is for example demonstrated by vague statements in politics and by humor based on wordplay. Even unwittingly, it is possible for statements to be understood differently. Such cases are commonly referred to as “ambiguities” and the result, when at least one understood meaning does not match the intended one, as a “misunderstanding”. The potential for ambiguities and misunderstandings raises the question in how far computational models of language should be capable of preventing, for example, users of voice assistants from being misunderstood or texts from being mistranslated.
In this talk, I will present a series of recent studies towards the automatic detection of potential sources of misunderstanding in instructional texts. I will argue that these instructional texts are, by virtue of their function, particularly suited to this task and I will show the extent to which potential sources of misunderstanding can be found through the revision history of such texts. Finally, I will discuss current results and findings, which may provide an outlook on how to account for misunderstandings in future NLP models.
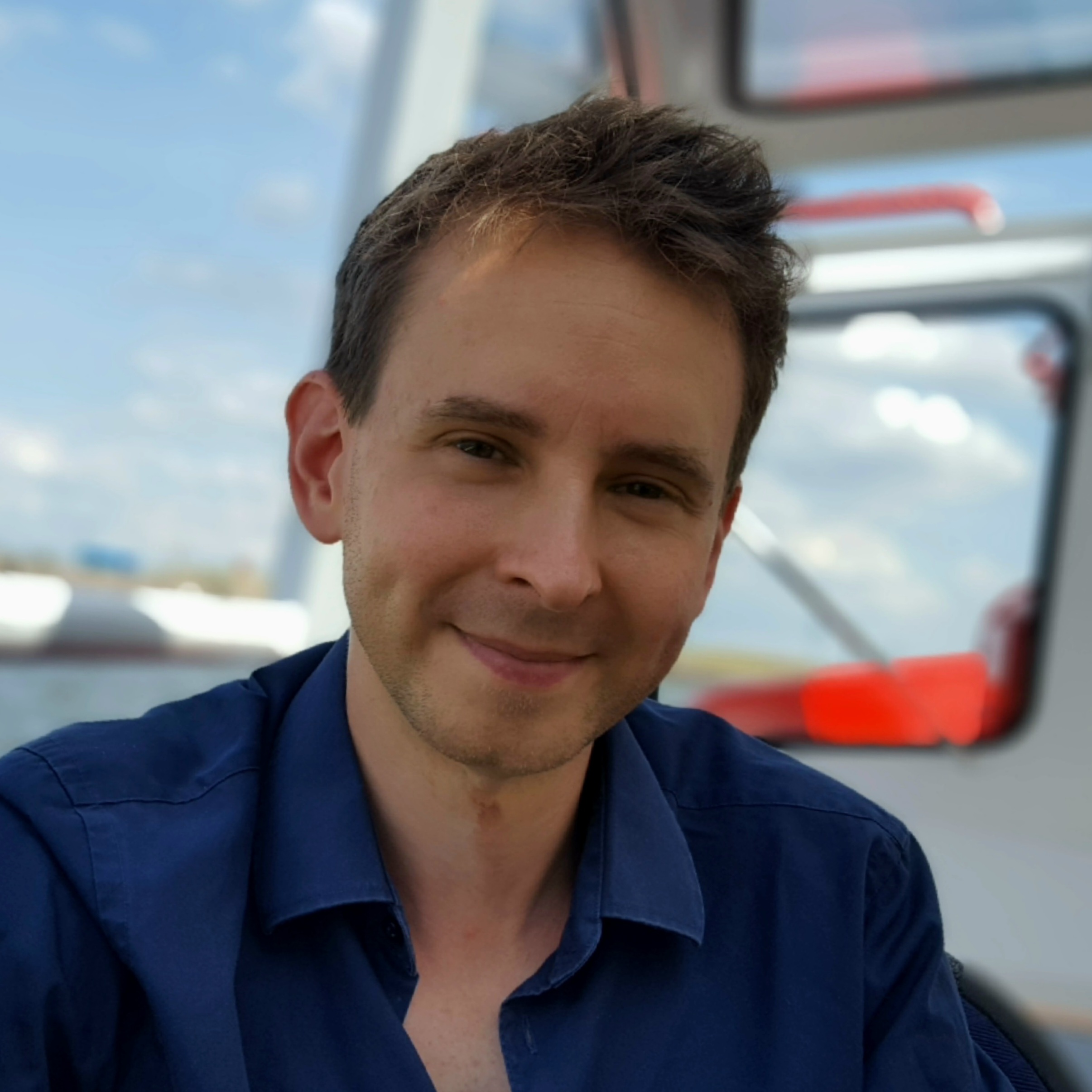
Biography
Michael Roth is an independent research group leader in the DFG Emmy Noether program. He studied computational linguistics at Saarland University and received his PhD from Heidelberg University in 2014. He then worked as a postdoc in Stuttgart, Edinburgh, Urbana-Champaign, and Saarbrücken, where he conducted research on models of lexical and role-based semantics, implicit meaning, and script knowledge. His current group is based at the University of Stuttgart and focuses on modeling sources of misunderstanding in complex instructional texts. Roth co-organized a number of workshops on semantics and commonsense knowledge, he is a regular area chair at *ACL conferences, and research in his group recently led to two best paper awards (at EACL-SRW 2021 and at SemEval 2022).